With the help of statistics, you can predict equipment breakdowns, predict how many customers will go to competitors, and find out which employees will leave soon
What is predictive analytics
Predictive (or predictive, predictive) analytics is a forecast based on historical data. Using statistical tools, you can identify patterns in changes in indicators in previous periods and predict how they will behave in the future. For example, by analyzing stock quotes, you can calculate a collapse or price change. Banks use predictive analytics when evaluating a borrower by analyzing financial performance and calculating the likelihood that the client will not be able to repay the loan.
Large companies create entire departments dealing with predictive analytics. They pursue different goals – from optimizing advertising costs to increasing production efficiency. Of all the types of business intelligence, predictive analytics is considered to bring the greatest benefit to companies.
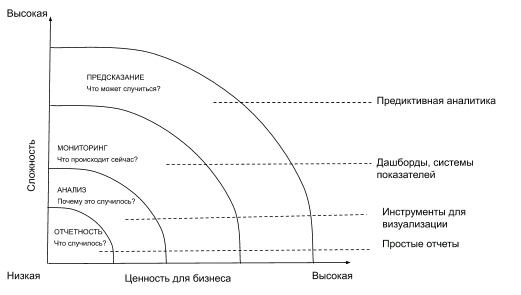
How the process is built
The basis of predictive analytics is big data. These are huge amounts of information that cannot be processed using the usual tools. Now IT companies offer ready-made programs that analyze big data and visualize them in the form of dashboards – visual tables, graphs and reports. The most up-to-date dashboard to date was created by the Center for Systems Science and Engineering at Johns Hopkins University. It shows the number of coronavirus cases in all countries.
Big data appears all the time – it is generated by companies, devices, and ourselves when we use smartphones and computers, shop and travel. In addition, they are easily collected and digitized: for example, if we used to buy products in the markets and pay in cash, now we often pay for goods with bank cards or place orders in an online store.
Common data examples:
- generated on the Internet – site traffic, data on purchases in online stores, “likes”;
- corporate information – transactions, reports on calls to the company, the number of customers;
- instrument readings – information from various sensors, telemetry data;
- economic indicators.
If these sources can already be called “classic”, then in recent years companies have learned to process less obvious data: the salaries of American football players, the content of films and the geographic coordinates of lightning strikes.
Building a forecast consists of several stages:
- Definition aims at analysis. This will depend on what kind of data you need to collect.
- Collection of data from various sources. To make a more accurate forecast, their purity and uniformity are important. Incorrect values can be entered in the process or software crashes, so it’s up to analysts to convert them into a suitable form.
- Analysis using statistical tools. There are ready-made solutions for this purpose, but some companies prefer to create software for their own needs.
- Modeling. At this stage, machine learning and other methods using artificial intelligence are often used. Analysts identify dependencies and factors that affect the behavior of indicators, and build a model with a forecast.
- Application in practice. This is the final stage, when it becomes clear how accurate the forecast turned out to be. During application, the model is trained on new data and corrects the forecast.
Predictive analytics cannot be 100% accurate. Otherwise, for example, the stock exchange would not make sense – everyone could predict how certain stocks would behave. In reality, every business metric is influenced by many factors, but the accuracy of the predictive model can be improved by working on the quality of the data and training it.
Examples of using predictive analytics
- Sales
Companies analyze the purchase history and current activity of the client. If, according to the results of the analysis, the buyer falls into the segment of those who can potentially go to competitors, then he can be offered a discount, bonuses or a gift.
- Personnel management
HR professionals use predictive analytics to predict in advance which employees will leave, which job applicants will succeed, how many positions need to be opened next year, how many employees will take advantage of different health insurance options, and so on. Google uses it to retain talent — if analytics predict a high-value employee is about to leave the company, they are offered a promotion or another position.
- Manufacture
By analyzing equipment usage data, you can determine when it will need preventive maintenance. So, in February, Mail.ru Group announced that it would create a digital platform for predictive analytics for Sukhoi. Data on the operation of industrial equipment and the parameters of operations will make it possible to predict the health of machines and carry out their timely maintenance.
- Finance and banks
In this area predictive analytics is used especially widely. For example, it is used to detect fraudulent transactions. Banks are looking at past years’ data on normal behavior: spending, typical times and geography of transactions. In case of anomalies, the organization receives a notification and can request additional confirmation of the transaction from the client.
- Marketing
Predictive analytics is especially effective in online marketing, where it is easy to collect information and make changes quickly. It helps to reduce advertising costs, show an ad that is relevant to a particular user, qualify a site visitor as a future paying customer, improve customer experience, etc.
- Epidemiology
In December 2019, BlueDot service specialists determined that the outbreak would be in Hubei province, publishing the first scientific publication that predicted the global spread of the virus.
There are unexpected applications for predictive analytics with artificial intelligence. Aleksey Sokolov, CTO of Redmadrobot Data Lab, spoke about them:
- Sports. ICEBERG analyzes hockey matches, collects statistics on players, their possession of the puck and predicts a number of indicators for clubs. Also in Japan, an algorithm was developed that predicts, based on the player’s posture, the place where the ping-pong ball will fall. According to the creators, the accuracy of the algorithm is 75%.
- Medicine. In addition to standard diagnostic tasks, predictive analytics is used for drug development (it can be used to model proteins for the treatment of certain diseases), the creation of individual treatment plans, and even for high-quality toothbrushing.
- Gambling. For a long time, poker was considered inaccessible to the machine. Now algorithms have learned how to bluff, predict the behavior of opponents and play better than the best players in the world.
“Systems built on machine learning are rapidly evolving. The main “food” for algorithms of this kind is data and computing power, and there are more and more of them. In five years, machine algorithms will permeate everything around just like electricity,” adds Alexei Sokolov. “Most likely, governments will learn how to correctly regulate smart technologies, unmanned vehicles will become the norm, and there will be breakthroughs in medicine that allow people to live longer.”
Subscribe and follow us on Yandex.Zen — technology, innovation, economics, education and sharing in one channel.