Contents
The Digital Breakthrough 2021 contest has ended. Its participants solved problems from the fields of ecology, urban security and housing and communal services using artificial intelligence. Trends offer to get acquainted with the most interesting cases
The final of the third season of the All-Russian competition “Digital Breakthrough – 2021” has ended. 251 teams took part in the competition, and hackathons were held in 14 regional IT hubs and in an online format. 36 best teams shared the prize fund in the amount of ₽24 million. As a result of the final hackathon, 232 solutions were developed for 12 proposed tasks, including in the field of ecology, industry, the development of unmanned vehicles and a smart city. Trends has collected the most interesting and unusual team decisions that involve artificial intelligence.
Prospects for digitalization
The European Investment Bank has conducted a study showing that the digitalization of business processes has a positive impact on the performance of enterprises in the EU and the USA. Analysts at the International Data Corporation predict that by 2022 up to 60% of global GDP will be created by digital companies, and the total investment in digital transformation in 2020-2023 will amount to $6,8 trillion.
In our country, the federal projects “Digital Technologies” and “Artificial Intelligence” within the framework of the national program “Digital Economy” are aimed at supporting the latest digital technologies – AI, the Internet of things, 5G, quantum systems and robotics. The developed solutions can be applied in different sectors of the economy. The authors of digital transformation solutions using Russian developments receive grants from the Ministry of Digital Development. In 2021 alone, ₽3,8 billion was allocated to finance such projects.
Oil spill monitoring
Rosatom invited hackathon participants to develop a system for detecting, assessing and monitoring oil spills using Earth remote sensing and machine learning technologies. According to the Ministry of Energy, in 2019 alone, more than 17 accidents with oil spills occurred at the enterprises of the fuel and energy complex, of which 10,5 thousand cases occurred at oil pipelines.
Rosatom provided the participants with a register of territories contaminated with oil and oil products in the regions of our country, as well as data from satellite images.
The best solution came from the Digital Coyote team. Their EcoTag system makes it possible to detect and analyze oil spills using machine learning. The peculiarity of the solution is that it stores all the collected information and generates accident reports by itself. The system uses images from space and data from oil spill sensors, a kind of buoys that have been proposed to be placed above the water.
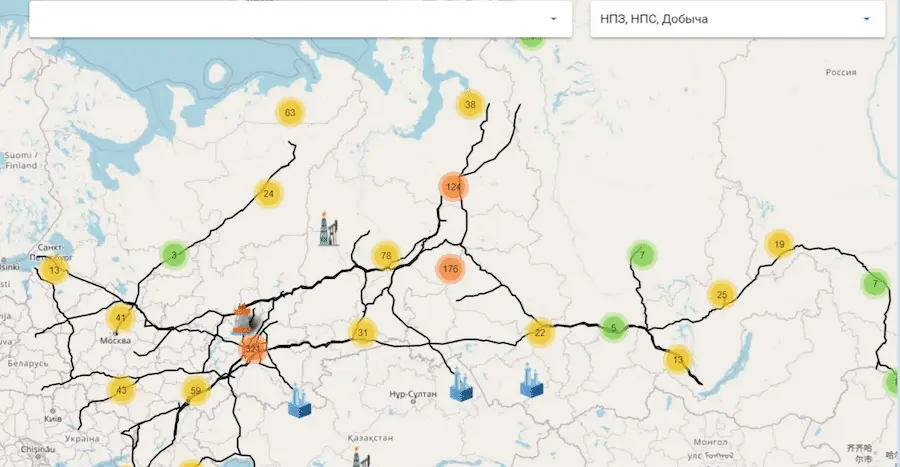
And the AIS team has developed a website that analyzes satellite imagery to detect contaminants. The model-based neural network examines images received through different channels and determines the presence of oil spills. She then prepares a report and exports it as a PDF. The neural network works on the principle of those used in text generation systems: it divides images into patterns, removes unnecessary ones, analyzes each pattern and correlates it with the location in the photo.
Vegetation monitoring in the area of power lines
The Ministry of Energy proposed to the participants of the competition to develop a system for calculating the area and height of vegetation in the protected zone where power lines are located. The agency noted that usually the work of assessing vegetation for clearing areas is carried out manually, therefore, in remote areas, it is associated with risks and monetary costs.
The automated system should use satellite imagery analysis. The hackathon participants themselves chose datasets and developed tools based on them. Another task of the system was the visualization of the collected monitoring results, including the determination of the height of vegetation.
The Digital Rover team became the winner of the hackathon. She proposed a solution based on Big Geo Data, which is based on a dataset of power lines covering the entire territory of the country. The system aggregates satellite imagery data from any free and commercial sources (Google, Yandex, Esri, Maxar) in real time. The model processes images and obtains data on both the area of vegetation and its height and type. Neural networks are used to more accurately estimate the height of plants. The database of images is updated automatically, which allows you to assess the situation in dynamics and predict its further development. The map is interactive, that is, you can navigate along it, and you can also use Yandex and Google maps as links for analysis.
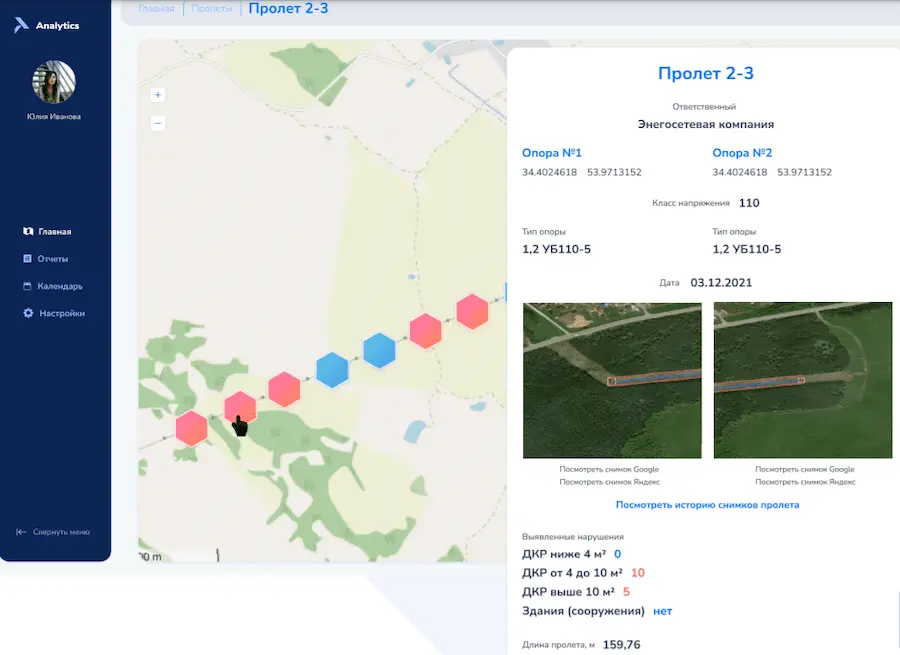
The Ezee team, which took second place, proposed a solution in the form of the Zeus AI system, which collects and processes satellite imagery from open sources to detect violations in the protected zones of power lines. The system allows you to automate monitoring of the entire perimeter of protected areas, and also determines the optimal cutting perimeters and logistics routes. Data labeling and the use of neural networks increase the accuracy of the system in time. When developing the system, the team marked up the data as follows: trees, grass, earth, shadow, buildings, water. Thus, the system can determine not only the height of vegetation in the power line zone, but also the shadow from it, which makes observations more accurate.
Assessment of the quality of raw materials in the field
Accenture offered to solve a case of checking for defects in raw materials that are unloaded in the field. The system should work in the absence of communication in the fields and is designed to fill the shortage of QCD workers.
The program must understand what and when happens to raw materials at the enterprise from the moment they are received and before they are transferred to production.
The Longterm_dev team has come up with a raw material quality analytics web platform that analyzes the condition of products upon acceptance through computer vision technology. The neural networks are trained on data about raw materials and containers so that they can reveal the percentage of defects in the images that come from the cameras.
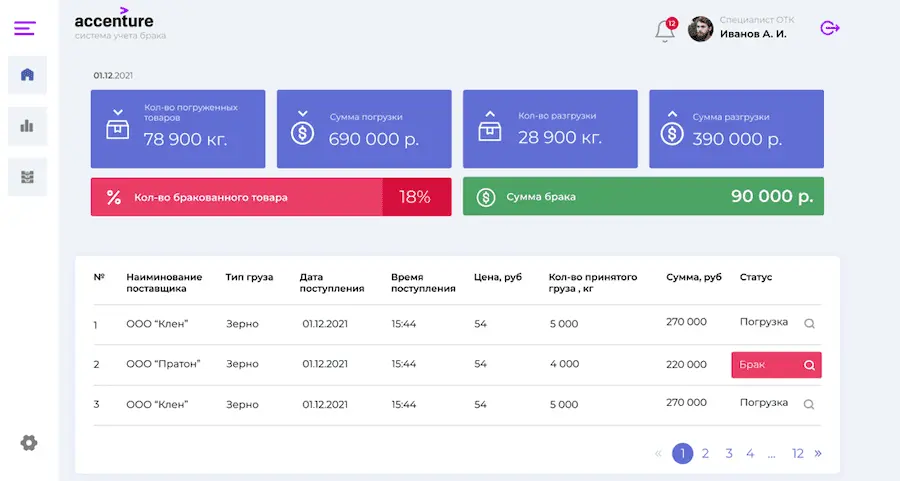
Online simulator for training drone operators
The Rosseti company presented a case that proposes to develop an online simulator system for training an operator of unmanned aerial vehicles. The hackers had to develop a prototype of such a system and a methodology for evaluating a specialist after he had been trained.
The AVM team has come up with a solution that will not only train UAV operators with theory, quizzes, and a VR simulator, but will also include an AI-based recommender system to tailor the learning path for the student individually, and automatically compose exam materials for the teacher .
Tracking garbage and accidents from city cameras
The Ministry of Digital Development, together with the authorities of the Republic of Tatarstan, offered the participants of the competition to create a service that will track garbage on the roads and the fullness of garbage cans according to data from city street video surveillance cameras. The system should have built-in IP cameras that are installed in the region as part of the “Safe City” concept.
The real-time solution must analyze the video streams or images from these cameras and then notify the appropriate services.
The Power Rangers DS team proposed a smart city system that will analyze video streams from city cameras, including five blocks: Clean Yard (waste management), Road Worker (road work management) TEPLO-MAPS (pedestrian traffic analysis ), “Parking” (fixation of violations of parking rules) and “SAFETY Park” (identification of violations of law and order). To use the system, it will be enough to connect any camera via IP and select the tool of interest.
Members of the UNI team have developed a platform for public utilities and residents, where users can mark a point on the map, describe the situation and send an appeal to public utilities, find out about the availability of parking spaces in the yard, and monitor the work of housing and communal services. The system is based on neural networks that analyze streaming information from cameras.
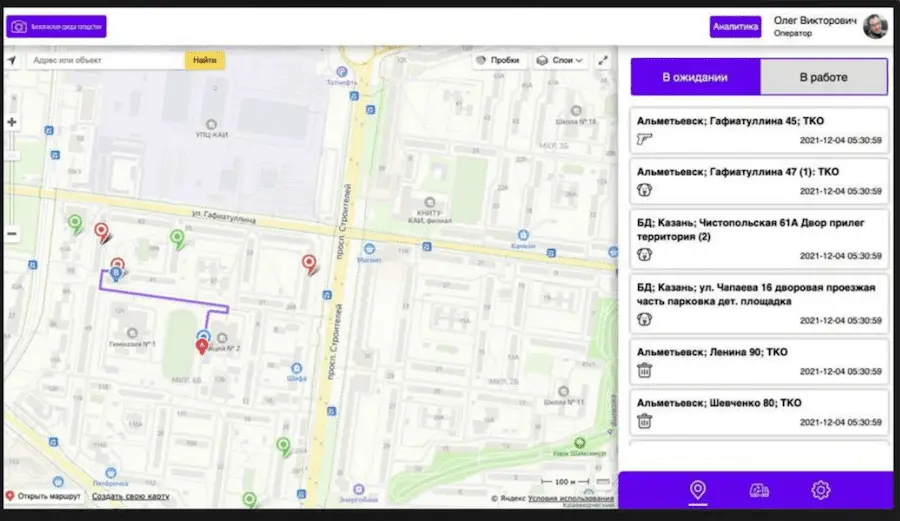
Create video recommendations
invited hackathon participants to develop a solution for converting video to text in the Xavier recommender system. The system provides each reader with personalized news, as well as video recommendations on websites and applications. The solution should improve the quality of selection of relevant content.
The Know What They Do team used existing machine learning models, including a solution from Nvidia. The system developed by the hackathoners, after loading a video into it, using one neural network, transcribes the text, extracts names and titles from it, and with the help of the second one, it determines and recognizes the text in the picture. As a result, the user receives more accurate recommendations for the selected tags, including even fragments of not completely relevant videos.
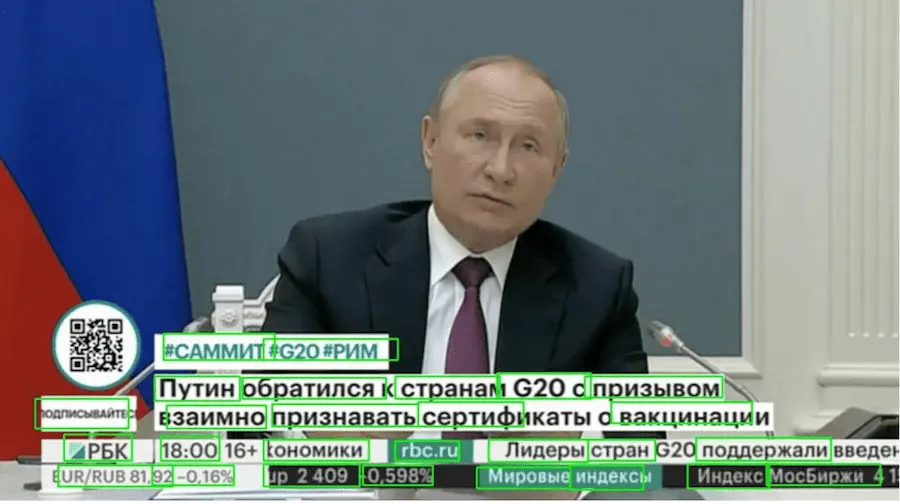
The HackTheHack team introduced a video information extraction module with speech-to-text mechanisms that corrects anglicisms, detects objects in the video, and looks for matches by names and titles. This system can be extended by adding new data. The hackathon participants used, among other things, the Russian-language GPT text generation model from Sberbank. The system also takes into account the emotional coloring of words. The developers noted that in the future it can be scaled by analyzing voices on video.