Contents
In order to track rare and endangered species of animals, you need to quickly receive data about them. Machine learning techniques help scientists solve this problem.
In 2019, the United Nations presented a report stating that about a million species of plants and animals, or 25% of the entire living world, will be endangered. There are more than 120 such species on the list of the International Conservation Union, and there is not enough information for their conservation for another 17. Researchers around the world are looking for ways to maintain biodiversity on Earth. In their work, in particular, they actively use artificial intelligence technologies.
How it works
Traditionally, animal observation has been based on fieldwork conducted by human experts. However, this work is time consuming, expensive and laborious. In addition, a person cannot penetrate everywhere, and his personal assessment may be biased. Finally, human presence can harm wild animals, their habitats, and be dangerous to observers themselves.
These limitations make understanding of geographic ranges, population densities and diversity much more difficult, leading to erroneous predictions and hindering rapid and effective action. For example, insufficient monitoring has led to misguided primate conservation strategies.
Machine learning offers an alternative to human research. Algorithms learn from data labeled with specific labels (animal species, etc.) and then make predictions based on the new data.
Population analysis by photo
The Translational Data Analytics Institute (TDAI) at The Ohio State University uses machine learning to facilitate species monitoring. They extract biological data from photos and videos.
The team has developed an open source platform called Wildbook that helps researchers collect and analyze photographs. She also trained the algorithm to examine traits of species, such as spot color or fin length. The algorithm scans publicly available images of a particular kind, using sources ranging from social media to digitized museum collections. The platform has already automatically analyzed 17 million images.
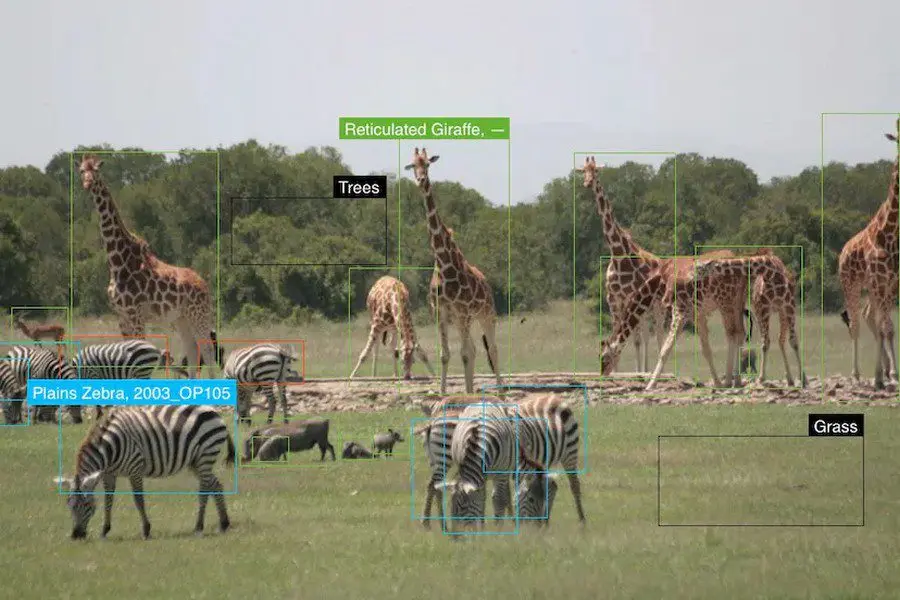
When analyzed, the AI can scan for details that humans would most likely not notice, such as the color scheme of the tiger moth’s wings. An earlier study showed that the human eye is unable to distinguish between the genotypes of male arboreal tiger moths of different ages.
The team implements algorithms that, based on the analysis of datasets, generate averages for various patterned animals such as leopards, zebras and whale sharks. They then analyze where those values change the most. This allows you to track animals non-invasively and without GPS collars, estimate population size, understand migration patterns, and much more.
The goal of the project is to help wildlife researchers overcome the lack of data and offer protection measures for endangered animals.
For example, the platform scanned 11 images of whale sharks to help researchers identify individual individuals and track their movement. This provided updated information on population size. As a result, the International Union for Conservation of Nature has changed the conservation status of the whale shark from “Vulnerable” to “Endangered”.
Now scientists have begun collaborating with researchers studying leopards in India to compare spot patterns between adults and their offspring using algorithms.
Discovery of obscure species
Researchers from the Norwegian University of Science and Technology, at the request of the International Union for Conservation of Nature, used machine learning to analyze what happens to those species of animals and plants for which they could not collect enough data for routine observation. They used information from 28 well-studied animal species. Experts have trained an algorithm to predict the extinction risk of 363 species for which there is insufficient data. The algorithm took into account the factors of human activity – for example, the production of hydroelectric power or plastic pollution.
The researchers found that 56% of these species are endangered. Amphibians turned out to be in the most vulnerable position – in this class, 85% of little-studied species may become extinct. The list includes the Malian squealing frog, spotted narrow-mouthed frog and other species. In 76% of cases, the predictions of the algorithm turned out to be correct.
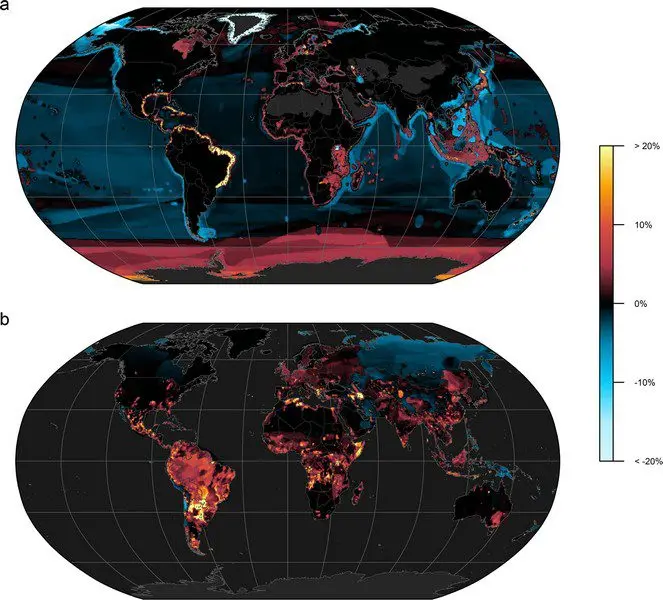
The authors of the work noted that their tool will provide an accurate and quick preliminary assessment for entering the species into the Red Book, facilitate this procedure and make it more objective.
Camera trap analysis platforms
Researchers are creating machine learning tools to analyze population data on a global scale and even involve ordinary volunteers in this process. For example, Wildlife Insights, a global machine learning platform, collects data on wild animal populations in real time for monitoring. It was developed by Conservation International, the North Carolina Museum of Natural Sciences, the Smithsonian Institution, the Wildlife Conservation Society, and Google.
Wildlife Insights was trained on data from the International Union for Conservation of Nature to monitor protected areas. It generates data from camera traps around the world.
With Wildlife Insights, researchers can access, share, and analyze global wildlife data to propose conservation actions.
Two main modules are built into the platform: the Wildlife Identification Service (WIS) and the analytics module. WIS runs on a neural network with a classifier pre-trained to embed images from Inception, a model that is widely used in image processing to identify objects. The model is trained on labeled data with time and geospatial labels. WIS has already processed 18 million of these images and is constantly retraining on new data.
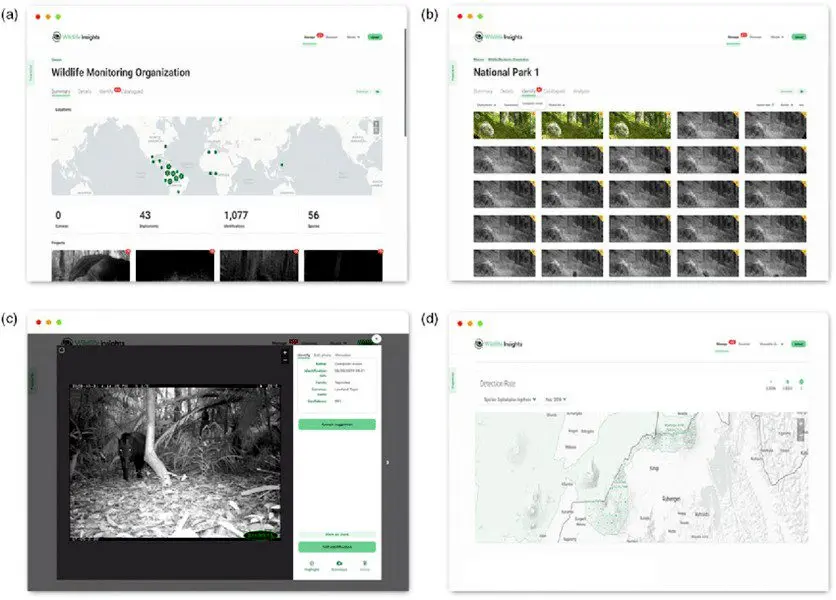
The information obtained through Wildlife Insights will allow you to track the state of protected areas and plan activities for more effective conservation of species.
And data company Appsilon teamed up with the University of Stirling in Scotland and the Gabon National Parks Agency (ANPN) in 2020 to develop the Mbaza AI image classification algorithm. It allows for the monitoring of biodiversity in Gabonese’s Lope and Waka National Parks.
Conservationists set up camera traps to take pictures of various species, including African forest elephants, gorillas, chimpanzees and pangolins. To train the algorithm, they used a large data set of more than 1,5 million images from several countries and from different camera trap models. After training, Mbaza AI was used to analyze more than 50 images collected from 200 such camera traps. It classifies up to 3 images per hour with 96% accuracy. Now conservationists can observe and track animals and quickly spot anomalies or red flags. The project is currently working with hundreds of camera traps.
Population Migration Tracking
In order to protect a certain species, you need to know the territory of its habitat. This allows the creation of protected areas. However, visually locating animals can be difficult, especially in difficult-to-reach environments. For example, humpback whales make characteristic sounds that can travel hundreds of kilometers under water. The National Oceanic and Atmospheric Association (Noaa) uses acoustic recorders in the Pacific Islands to monitor populations of marine mammals in hard-to-reach places. Over 14 years of observation, the researchers have accumulated about 190 hours of acoustic recordings. They then decided to use Google AI to use machine learning to recognize the humpback whales’ calls.
The developed tool made it possible to identify the songs of humpback whales in the data set and to identify patterns of their migration in the area of the Hawaiian and Mariana Islands. The scientists also found that marine mammals swim up to Kingman Reef, where their presence had not previously been noticed. The model was able to accurately locate the whales 97% of the time. Now researchers can evaluate how whale migration routes have changed over time under the influence of anthropogenic factors.
Animal Behavior Tracking
Information about how behavior changes within it helps to study the state of a population. Researchers from the Center for Advanced Research in Collective Behavior at the University of Konstanz and the Max Planck Institute for Animal Behavior have developed a machine learning tool, DenseNet, that can track changes in animal body position with high speed and accuracy. The algorithm is able to correctly measure the position of animals in images after being trained on just 100 examples. DeepPoseKit can be used to study wild animals in difficult field conditions.
Studying the behavior of species will allow researchers to learn how a population lives and develops, as well as how it interacts with the environment. The tool can be used to study species ranging from flies, locusts and mice under controlled laboratory conditions to large mammals in the wild.
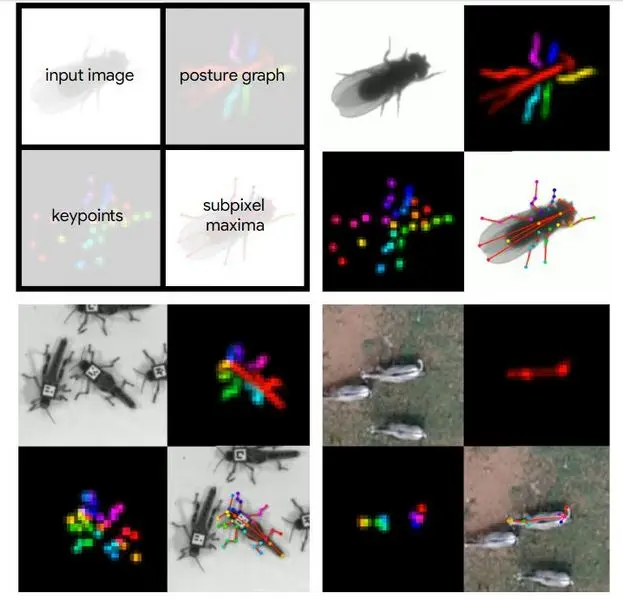
There are more and more such tracking tools, which makes it easier to remotely track the behavior of animals.
Moreover, machine learning tools are used to individually identify animals in a population. For example, researchers at Kyoto University and the Bossou Archive project applied facial recognition techniques to a data set of 1 million images of 23 chimpanzee species in Guinea. Recognition accuracy exceeded 90%. The algorithm successfully recognized, in particular, the gender of the chimpanzee. The authors of the development note that this knowledge will automatically track the natural demographic processes in wild populations. So, scientists have found that since the end of 2003, the size of the chimpanzee community has declined sharply due to an epidemic that caused significant demographic changes, and now it is rapidly aging.

Now the researchers intend to improve their system so that it can identify and track the life of individual individuals even on raw streaming video, that is, directly from cameras. This will help conservation, as it will allow us to analyze key events in the history of the wild community.
November 22 will be the RBC.Tech forum about technologies that change business. Leading experts from various industries will share their experience in implementing innovations, as well as talk about technological tools for solving specific business problems.