Contents
Artificial intelligence as an operator of automated processes is no longer a trope in science fiction, but a reality. He also quickly learns to do creative work traditionally considered the lot of homo sapiens.
What will a person do if robots supplant us in creativity as well? And is it worth it to be afraid at all?
News about the successes of robots and artificial intelligence (AI) is increasingly similar to summaries of combat losses. So, “Sberbank” is already threatening to greatly reduce the legal departments at the expense of automatic assistants built on neural networks. Most recently, MTS launched a robot lawyer on the market, and China launched an online court in 2017: a digital judge has replaced real lawyers and has already considered more than three million civil and copyright cases.
And if workers of even such an intellectual profession are being replaced, then there is nothing to say about work that does not require analytical skills: Yandex has already launched its own postman robot, and Amazon has been expanding its drone delivery technology for more than a year.
And yet, so far, automation concerns assembly line production and workplaces that perform a range of the same tasks. But what will happen when digital services are not limited to games with neural networks, but will actively use AI to work with creative content? Do artists, screenwriters and musicians have a place in the museum?
The Fourth Law of Robotics
Neural networks have long been used in big data analysis and the humanities, using machines to study slavery in 1840s Texas, rap artists, and assist in precision farming. But when it comes to the production of a creative product, the tasks of AI are not limited to analytics. Strictly speaking, there are two groups of tasks here: to assist creative professionals in working on a work; to take on routine tasks and leave a person more time to develop a general concept. Or, in fact, create a work based on the downloaded data. Both areas are developing rapidly.
So, in 2018, the American writer Robin Sloan shared his experience of co-creation with a neural network. Sloan worked like this: he wrote part of the sentence, and then clicked on the button so that the neural network, built on the open GPT-2 model, would complete the sentence itself, using the context and the data loaded into it earlier. Previously, Sloan collected a whole corpus of texts that he “fed” neural networks: it included novels by John Steinbeck, Joan Didion and Philip Dick, stories by American science fiction writers, interviews with developers from Silicon Valley, and poetry by Johnny Cash. Sloan himself is not a techno-optimist and does not think that AI will ever write novels for people. It is just a tool, not a replacement for a person.
“If you weigh the pros and cons, people will still prefer to read texts written by a person with real life experience who writes about the reality of human existence,” Sloan said.
Worthy of mention are several more cases of co-creation of a computer and a writer. For example, in 1993, for Just This Time, Scott French created a special program to emulate the style of popular romance author Jacqueline Susan, and Botnik Studios experimented with Harry Potter fan fiction written by a neural network.
But AI isn’t just helping writers now. In 2016, Sony launched the Flow Machines neural network assistant, which is able to offer musicians ready-made arrangements and melodies based on already written music. Back in the nineties, David Bowie helped develop Verbasizer, a random word generator for songs. And artists have long been using neural network algorithms to correct defects in their work and apply ready-made models.
But a robotic assistant is one thing. What about a robot creator?
Portrait of an algorithm artist
Here, too, AI artists were the first among the robots-producers of content, whose works managed to turn into a separate genre. Very soon, neural networks will be able to “bury” the traditional profession of a colorist for the comics industry: the beta development of the Clip Studio program from Celsys can automatically overlay colors on a black and white drawing. Anime studios and manga publishers are already interested in the algorithm, and artist Joao Do Lago, who worked, among other things, on the Castlevania anime series for Netflix, believes that automatic colorization will free up a lot of efforts from artists who previously had to spend a lot of time creating a recognizable and at the same time, the unique visual style of the works.
But robotic artists have gone beyond being a colorist. In October 2018, the first portrait painted by a neural network went under the hammer: “Portrait of Edmond Belamy” was sold for 432 thousand dollars – the price was ten times higher than the declared cost of the painting.
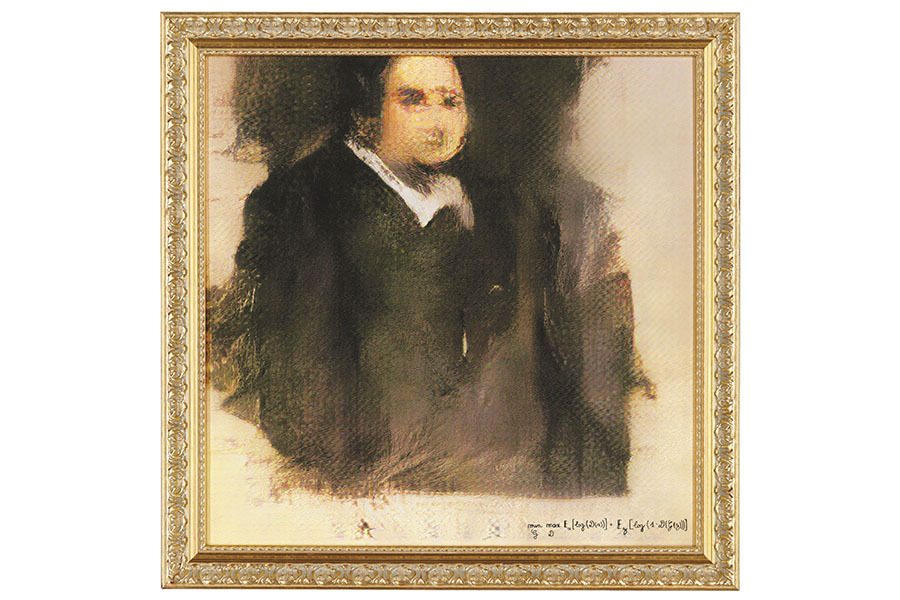
Text-generating machines are not far behind. In May 2020, OpenAI introduced the third version of the GPT text generation algorithm, which was trained on 175 billion parameters. A Reddit user accessed GPT-3 a few weeks ago and generated two short stories in the style of Neil Gaiman and Terry Pratchett. Journalist and media expert Alexander Amzin translated the story in the “Gaimanian style” because “when I read it, it became really scary.” According to Amzin, in a few generations the algorithm will learn to generate “quite interesting texts.”
And a couple of years ago, a neural network built jointly by Yandex and Kinopoisk wrote a rather frightening story based on the works of Nikolai Gogol. Back in 2016, the same algorithm wrote the album “Neural Defense”, based on the melody and lyrics of the legendary band Yegor Letov.
Yet the development of neural networks has a significant limitation. It has to do with computing power. Researchers at the Massachusetts Institute of Technology analyzed more than a thousand scientific publications on the topic of deep learning. Particular attention was paid to such applications of technology as image classification, object detection, question answering, named entity recognition, and machine translation. For each publication, the number of computational operations and the amount of power required to create a model of a certain accuracy were estimated. It turned out that even with a significant increase in computing power, the accuracy of modern models increases slightly, and a larger increase in power would require huge amounts of money and a serious burden on the environment.
Should we expect scripts from robot writers and paintings by digital artists? This seems to be a very distant prospect so far, the realization of which is directly related to the ability of technology companies to provide resources for new self-learning systems. And although robots are already capable of generating “formula” (that is, based on a ready-made plot scheme) stories at a high level, their creativity is still subject to the intention of the person behind the keyboard.
If we imagine the robots of the future, which, as in the novels of Viktor Pelevin, create their own texts, then we can assume that in this case the authorship will simply go “level” higher: the fees will be received not by machines, but by people working with them or entire companies. . This is exactly what is happening with AI artists now. The real creativity in machines is still far away.
“It is too early for artists to change jobs”
Pavel Osinenko, Senior Lecturer at the Center for Scientific and Engineering Computing Technologies for Big Data Problems at Skoltech CDISE, comments:
Any question from the category “can AI replace a person in such and such?” one way or another comes down to the question: “Does natural (human) intelligence have some non-algorithmic nature, which cannot be implemented in principle in a computing device?”. “Realize” – in the sense of reproducing a function identical to that of natural intelligence.
The question may seem somewhat far from science and technology and rather close to philosophy, but one way or another, any chain of argumentation will lead to it. Therefore, taking one or another point of view on it, we will come to the conclusion that either a person is fundamentally irreplaceable in a particular area, or any function of natural intelligence is fundamentally reproducible in a computing device.
Our understanding of how the brain works is constantly improving, and only in the last decades has tremendous progress been made. Let’s say we have a better understanding of how synaptic connections form certain associations. The direction of brain modeling is actively developing: for example, the Blue Brain Project recently announced a simulation of a fragment of a rat “gray matter”.
And some go so far as to consider the entire universe to be the result of a calculation. This point of view was expressed by Elon Musk and physicists Stephen Wolfram, Max Tegmark. Indeed, how can one explain such an unprecedented success of computational mathematics in describing and transforming the world? Of course, these arguments, at least for today, are philosophical in nature. I personally do not believe in the existence of some incomputable element of human consciousness, in connection with which I believe that, in principle, any function of natural intelligence, no matter how high-level or, if you like, highly spiritual it may be, is transferable to a digital medium. The question here, rather, is scaling and complexity.
And here we come to the next problem. There are more or less strictly derived, fundamental limits on which of the calculations are in principle realizable in the Universe. Here we can mention, say, the Beckenstein limit. Theoretically, of course, there are limits to computation. But they are so conservative that, from a practical point of view, they are unlikely to interfere with modeling, say, the human brain. Some technical limits are more relevant. For example, the density of transistors in a processor. Moore’s law has been buried more than once, but so far, apparently, it’s too early. At the same time, Google recently confirmed the achievement of quantum supremacy, which will be a significant milestone in the development of quantum computers.
Yes, deep neural network computing is heavyweight, and sometimes researchers focus more on the growth of computing resources than on optimizing algorithms. It’s interesting to note that reinforcement learning, the vanguard of machine learning, seems to be a very good candidate for quantum computing, and the “limit of machine learning” is probably not to be taken too literally.
In conclusion, answering the question: “Is it time for artists to look for a new job?”, I would say: “You can think about this at your leisure, but in the coming decades you can probably not strain yourself.”