Contents
AI technologies are still mainly used in experimental farms. Despite the possibilities of precision farming, early detection of pests and diseases, for most farmers they remain expensive and redundant.
Agriculture is a rather conservative and inert industry. Little has changed in the cultivation of cereals for centuries after the innovations of the horse-drawn plow and three-field. For example, in viticulture, everything is preserved approximately as it was 4-6 thousand years ago.
Cardinal innovations happened relatively recently, in the XNUMXth century: the introduction of agricultural chemicals (herbicides and pesticides), the emergence of hybrid varieties of cereals (primarily corn, which is present in one form or another in almost every product of deep processing in the United States), electrification, artificial insemination, agricultural machinery.
In the early 2000s, it seemed that the potential of these innovations to further increase labor productivity in agriculture was exhausted, progress was slowing down. When several people are constantly working in production, there is no time left for experiments. Since then, farmers, who produce up to 80% of all agricultural products in the world, have been persistently trying to make friends with advanced technologies: nano, bio, smart and AI.
There are about 1 startups in the “agriculture and farming” direction in the Crunchbase list, tens of thousands of names in IT. In agribusiness, you have 300 attempts to test if a hypothesis works while you are conditionally between 40 and 20 years old; in technology, you can make 60 attempts per week. About 40 startups in the field of smart farming, precision farming, and biotechnology are represented on the Russian market.
Global spending on smart technologies in agriculture, systems based on artificial intelligence by 2025 is projected to triple compared to 2020, reaching $15,3 billion. Farm spending on technical solutions with artificial intelligence alone will increase from $1 billion in 2020 to $4 billion in 2026 (data from Markets & Markets).
What can AI do in agriculture?
The argument in favor of the massive introduction of AI technologies in agriculture is often formulated as follows: the human population will reach 2050 billion people by 10, it is impossible to radically increase the cultivated areas, it is necessary to increase the intensity of their use. Imposing on farmers the task of feeding the entire population of the globe is like asking medium and small technology companies to solve the problem of global warming.
At the moment, producers and consumers agree on one thing – the desire to make quality agricultural products more affordable. Are modern technologies able to solve these purely utilitarian questions?
By AI technologies, although in the strict sense, artificial intelligence is unlikely to appear in the foreseeable future, we will mean, for ease of classification, a set of solutions in the field of machine learning and Big Data processing, neural networks, machine vision, and so on.
In the context of the use of AI in the cultivation of grains, vegetables and fruits, and animal husbandry, there are three key areas of how this can work in theory in principle:
Early detection of pests, diseases and weeds
Problem. Today, farmers manually inspect each section of their field for “malfunctions”, visually inspect the condition of the herd. Resources and experience may simply be lacking to detect diseases in the early stages.
Solution. A drone equipped with computer vision that regularly monitors the plot (change in color of a leaf or ear can serve as a signal) or herd (changes in weight can be monitored).
Example: Salmon farms in Norway use stereoscopic cameras for early detection of sea lice disease in fish. The disease causes hundreds of millions of dollars in losses every year. The Norwegian government plans to make the technology an industry standard.
Precision farming
Problem. Farmers apply fertilizers and irrigate with a continuous carpet, although areas of the same field may have different conditions, topography, soil composition: that is, somewhere there will be a shortage of resources, and somewhere there will be an oversupply.
Solution. Sensors connected to the Internet of Things (IoT) network monitor the main indicators: soil moisture, temperature, site illumination, which are necessary for optimal farming. The algorithm makes recommendations for each square meter of the field, which leads to savings in water, seeds and chemicals.
Example. Several “AI farms” are located in China’s Guizhou province, which has cheap labor and a climate that allows machines and data centers to operate without expensive cooling systems.
Yield calculation
Problem. It is extremely difficult for farmers to predict the result of their efforts to grow grains, vegetables or fruits year by year, especially when it comes to introducing new varieties, pesticides and so on. In general, many factors affect the yield.
Solution. Information collected by sensors or drones is analyzed by machine learning algorithms that operate, among other things, on historical data on climate change, field maps are created, and patterns are identified. As a result, the farmer can calculate the yield from each plot and even the change in the price of his products, optimize the use of resources.
Example. Digital platform for precision farming in Argentina. The system uses machine learning, drone and satellite geodata, and cloud computing to develop recommendations for farmers in real time.
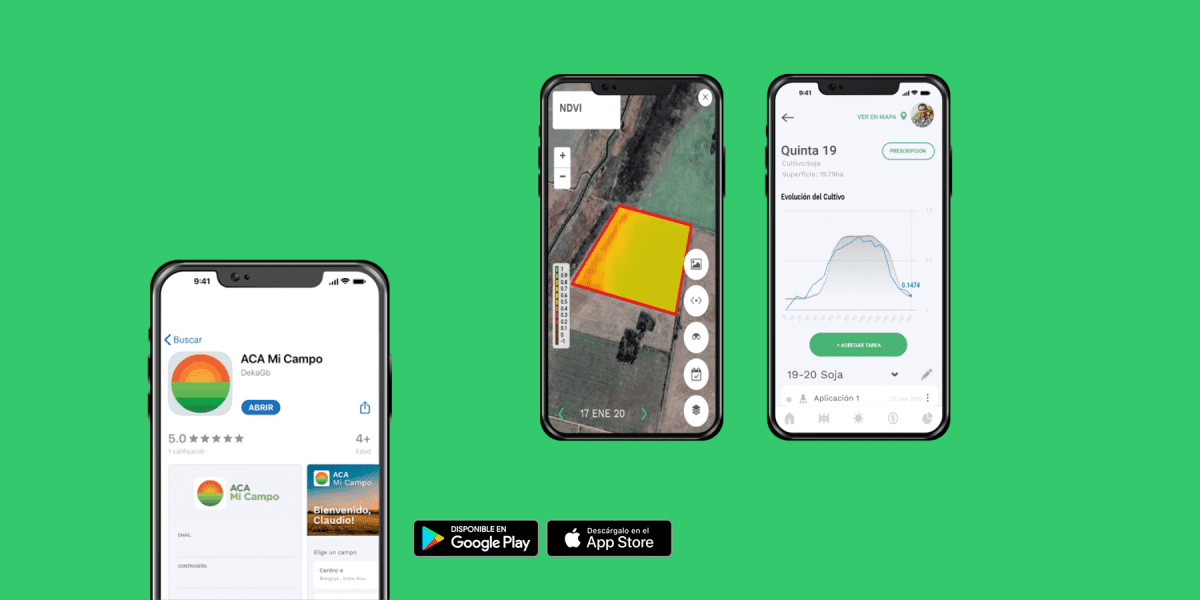
Urban farming can be singled out as a separate example. An Israeli tech company used AI algorithms to find optimal lighting and humidity conditions to grow agricultural products in small home containers.
Where else is there evidence that this is implemented and working?
There are very few cases of application of AI technologies by farmers in open sources. Almost all information comes from the developers of such solutions in the context of their potential.
Let’s take a step back: from AI algorithms to simple automation. Fully automated, with minimal human intervention, plowing fields and harvesting grain does not seem like a difficult solution. The trajectory of movement here is simple and clear. All that the driver of the equipment does when preparing the field is a U-turn. There are no obstacles in the form of other vehicles or people.
The first concept of a fully autonomous tractor (without an operator in the cab) was released by CNH Industrial in 2016 in two versions at once. So far, there is no information about the launch of production and the mass introduction of the model in agricultural complexes.
John Deere, the largest agricultural machinery manufacturer in the United States, which also already presented the concept of a tractor with an autopilot at one of the exhibitions, only in August 2021 announced the creation of a startup called Bear Flag Robotics, which is going to bring the technology to perfection. In fact, the company admitted that there is nothing similar on the market now.
In terms of the development of AI technologies in agriculture, we are talking about the point use of sensors and drones in experimental farms. These are isolated examples across the industry. It is too early to talk about the widespread introduction of such solutions by farmers.
Objective obstacles
The interest of farmers in the latest technical solutions in agriculture is still there. For example, large agricultural complexes in China during the period of the spread of African swine fever tried to introduce machine vision to identify and isolate sick individuals. The problem is that small farms, which are the majority, simply cannot afford it yet.
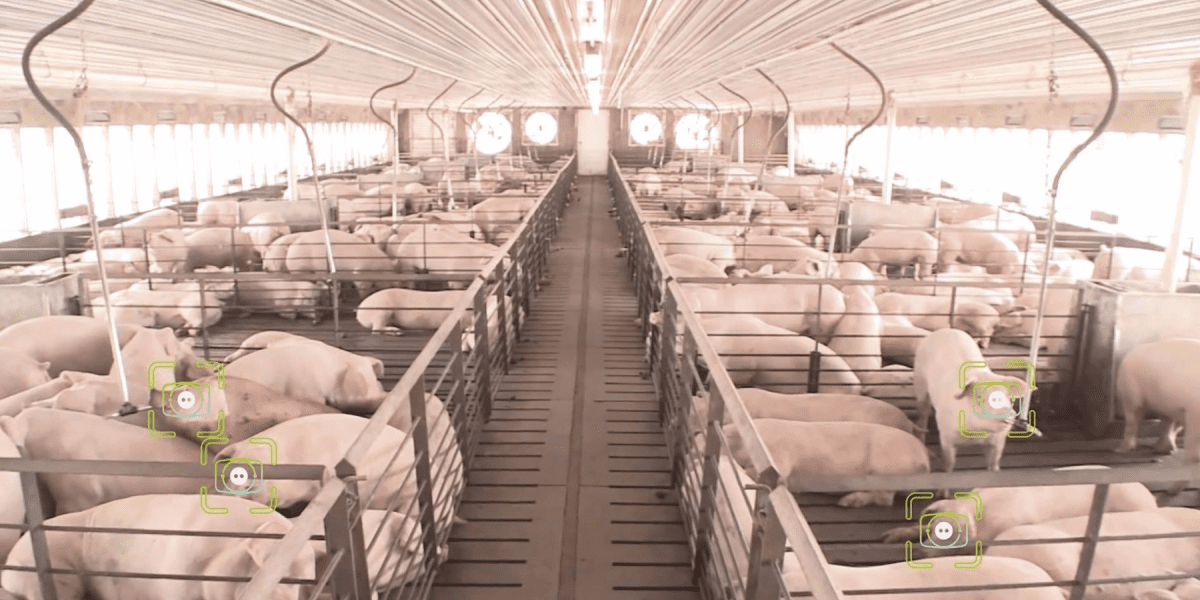
AI technologies for the mass agricultural producer are expensive and redundant. Rural areas have cheap labor. Plus, there is a social context: to free even those few workers, replacing them with robotic technology, is a social problem.
In addition, as we know from the example of Russian farms, an increase in yields does not make farmers and agro-complexes richer, it simply leads to a collapse in purchase prices from intermediaries. In such a situation, it is difficult to find an incentive to introduce costly innovations. Despite the fact that the farmer, as a rule, does not have free funds that could be invested in large-scale technical re-equipment.
The objective obstacle is that in agriculture there is a really long cycle of hypothesis testing and development of new technologies. At an experimental enterprise, in a field laboratory, it is difficult to simulate conditions that would be suitable for other regions, with a different climate, humidity, topography, and soil composition.
The implementation of AI-based solutions requires basic technical and digital equipment. Connecting sensors to an IoT network simply requires stable high-speed Internet coverage. Data must be processed and stored somewhere. This means that you need to look for computing power or build your own local data centers. For UAVs (drones), restrictions on use are established by law.
Widespread adoption of technology carries its own risks. Training one such system is an energy-consuming process. According to experts, it leaves a carbon footprint equivalent to 284 tons of CO2. That is, the launch of one AI system will cost the planet five times “more expensive” than the contribution to global warming of one car.
AI should not be viewed as a “silver bullet” for solving all the global problems of the industry. But this is one of the promising areas, certainly worthy of attention.